Abstract
This article describes the development of a representative dataset of extractables and leachables (E&L) from the combined Extractables and Leachables Safety Information Exchange (ELSIE) Consortium and the Product Quality Research Institute (PQRI) published datasets, representing a total of 783 chemicals. A chemical structure-based clustering of the combined dataset identified 142 distinct chemical classes with two or more chemicals across the combined dataset. The majority of these classes (105 chemical classes out of 142) contained chemicals from both datasets, whereas 8 classes contained only chemicals from the ELSIE dataset and 29 classes contain only chemicals from the PQRI dataset. This evaluation also identified classes containing chemicals that were flagged as potentially mutagenic as well as potent (strong or extreme) dermal sensitizers by in silico tools. The prevalence of alerting structures in the E&L datasets was approximately 9% (69 examples) for mutagens and 3% (25 examples) for potent sensitizers. This analysis showed that most (80%; 20 of 25) E&L predicted to be strong or extreme dermal sensitizers were also flagged as potential mutagens. Only two chemical classes, each containing three chemicals (alkyl bromides and isothiocyanates), were uniquely identified in the PQRI dataset and contained chemicals predicted to be potential mutagens and/or potent dermal sensitizers.
- Dermal sensitization
- Mutagenicity
- Extractables and leachables
- Computational assessments
- Chemical class analysis
1. Introduction
Extractables are chemical entities released from a product contact material or component upon exposure to solvents of varying pH and polarity under exaggerated conditions of time and temperature (1). On the other hand, leachables are chemical entities that may migrate into a finished drug product from a product contact material under typical process or storage stability conditions. Given the potential occurrence of leachables in finished products, it is important to understand their toxicological relevance and determine if they could compromise product safety or quality.
In silico methods can support such a hazard assessment. Although there are currently no published guidelines on the methodologies to conduct an in silico toxicological assessment of extractables and leachables (E&L), industry practice commonly focuses on the assessment of mutagenicity and dermal sensitization (2⇓⇓–5).
The Extractables and Leachables Safety Information Exchange (ELSIE) Consortium and the Product Quality Research Institute (PQRI) both seek to advance the science around E&L risk management and provide recommendations on best practices in this area (4⇓⇓–7). These efforts have had a significant impact and have resulted in recommended practices for general identification and qualification of E&L (4⇓–6). Of relevance to this project is the publication of datasets by both ELSIE and PQRI, which offer a unique opportunity to investigate and better understand the E&L chemical space. Further, given the use of in silico models for mutagenicity assessment (8), and the considerations given to the weight of evidence provided by dermal sensitization models (7), the datasets offer the opportunity to also evaluate the in silico model prediction space across the E&L datasets. Understanding the chemical classes that are likely to be flagged by predictive models across the different endpoints adds value to E&L risk management as such an understanding can influence material selection and allow more rapid and meaningful qualification of E&L compounds.
The ELSIE database was first published in 2011 (9) and is described further by Parris et al. (5). It is a database of observed E&L that was compiled based on industry feedback. This database has been utilized to develop and evaluate data-driven approaches to the toxicological evaluation of E&L. For example, Broschard et al. (2) referred to the ELSIE database and the corresponding safety information housed within it to build case studies that demonstrate the applicability of key steps in the toxicological evaluation of E&L. Broschard et al. (2) also described the use of in silico models for mutagenicity to be employed in the absence of experimental data, in alignment with the International Council for Harmonisation of Technical Requirements for Pharmaceuticals for Human Use (ICH) guideline M7 for assessment and control of deoxyribonucleic acid (DNA) reactive (mutagenic) impurities. Additionally, Broschard and co-authors (2) also suggested that in silico methods and read-across approaches may support the risk assessment of E&L in this context. Parris et al. (5) conducted an analysis of the ELSIE dataset with the aim of understanding the prevalence and scope of potent (strong or extreme) dermal sensitizers in the E&L chemical space. Potent dermal sensitizers have been identified as being of more concern than weak or moderate dermal sensitizers given the potential for adverse effects at low exposures (low exposures are more consistent with anticipated levels of leachables) (5).
In order to assess exposure-based thresholds, PQRI established a list of chemicals that were known to extract or leach from manufacturing equipment as well as container closure systems for parenteral drug products (7). The dataset is representative of typical polymers used in pharmaceutical and medical device components (e.g., containers, closures, adhesives, and coatings), manufacturing components (e.g., bags, tubing, and connectors) that encompass classes of various thermoplastic and thermoset polymers (e.g., polyolefins, polyvinyl chloride [PVC], polyurethanes, polyamides, polyesters polyacrylates, silicone, and synthetic isobutylene or isoprene elastomers), as well as ingredients that are intentionally added (e.g., antioxidants, fillers, and colorants). Polymerization is a chain reaction process that involves a monomer addition or condensation reactions and cross-linking compounds in the case of thermosets. The sources of extractables can esult from residuals of the polymerization process (e.g., monomers, solvents, surfactants, and catalysts), or the forming and assembly processes (e.g., mold release, lubricants, or stabilizers). Although not directly related to the starting materials, post processing (e.g., washing or sterilization) and aging are other sources of extractables. The PQRI extractable database is representative of a variety of chemicals used for multiple purposes that can occur throughout the life cycle of the material, starting with the initial polymerization to storage of the final components over time (e.g., silicone, fatty acids/amides, oligomers, low-molecular-weight polymers and degradation products, as well as various plasticizers).
This paper outlines the development of a representative dataset of E&L from the combined ELSIE and PQRI published datasets. Combining these datasets and understanding the structural classes contained within each source will allow us to establish whether additional unique classes from such sets would add value to a previous analysis that focused on a single dataset (5). The combined set also offers the advantage of increased structural diversity and broader representation of the E&L chemical space. Therefore, an analysis of this combined dataset was performed to help understand the structural diversity of this chemical space and identify classes of chemicals that are likely to trigger in silico alerts for mutagenicity and dermal sensitization. This work builds upon the framework for sensitization hazard assessment discussed by Parris et al. (5) and the importance of gauging the prevalence of strong or extreme (i.e., potent) dermal sensitizers in the E&L chemical space.
2. Methods
The following sections describe the methods that were used to combine the ELSIE and PQRI datasets, identify chemical classes and assess the prevalence of mutagenic and potent dermal sensitizers in the combined dataset.
2.1. Datasets
The ELSIE and PQRI published datasets were used to develop a combined dataset which was used for further analysis.
2.1.1. ELSIE Dataset:
The ELSIE dataset is composed of 466 compounds (before conversion to the structure–activity relationship [SAR] form [described later]). Compounds observed in pharmaceutical, biological, device applications, and processes were compiled into a dataset (elsiedatabase.org) (5). The ELSIE database was established because ELSIE consortia members wanted to reduce duplicative toxicological testing on individual chemicals observed as E&L by sharing safety information and data. The ELSIE consortium collects and populates the ELSIE database with chemicals that members have identified or observed as E&L. The database may also include compounds identified as additives that run a high risk of showing up as an E&L (but are not yet observed as such).
2.1.2. PQRI Dataset:
The PQRI set of structures represented 611 chemical structures (before conversion to the SAR form [described later]). The PQRI dataset includes compounds that have been known to extract or leach from manufacturing equipment and/or container closure systems during the manufacture of parenteral drug products.
2.1.3. Combined ELSIE and PQRI Dataset:
Both the PQRI and ELSIE datasets cover various commonly observed E&L as well as use categories and structural classes. These datasets can be considered as representative of the E&L chemical and functional space (10). The ELSIE and PQRI lists of chemicals were mapped according to their chemical structures based on the available CAS numbers using Leadscope’s internal chemical registration process (11). According to best practices in the field of computational toxicology, the chemical structures were converted to their SAR forms. In this process, salt forms are neutralized and complex structures, such as mixtures and polymers, are excluded from the set. When a counterion is removed and the compound is neutralized (as occurs in the process of creating SAR structures for organic salts), several organic salts (non-SAR parents) may be mapped to the same SAR form. Such structures were considered duplicates and were therefore represented by a single structure. For example, the chemical with identification number LS-179914 was presented in the calcium form. Conversion of LS-179914 to its corresponding SAR form results in the removal of the calcium ion and neutralization of the phosphonate anion. The resulting parent structure is identical to another chemical (LS-5672), which is already represented in a SAR form in the dataset (Figure 1). After SAR conversion, duplicate structures were removed from the ELSIE and PQRI datasets, resulting in a contribution of 413 compounds from the ELSIE database (466 originally) and 589 structures from the PQRI database (611 originally).
Illustration of the structure-activity relationship (SAR) conversion process for salts.
2.2. Analysis of Chemical Classes
The following section outlines the methodology used to group the chemicals. These groupings are used to help understand the chemical space for E&L and which chemical classes are likely to be flagged by in silico models used to assess E&L.
A dictionary of over 27,000 unique predefined named substructure queries (12) was used to support the grouping of the combined ELSIE and PQRI dataset. This dictionary of substructure queries covers a variety of organic structural classes including substituted benzenes, carbocycles, functional groups, and heterocycles as well as a variety of additional chemical moieties. In Figure 2, two chemical structures are shown to illustrate the matching of two different structural features from the dictionary: (i) “alkene, 1,1-disubst” and (ii) “alkene, monosubst”. Each substructure query is precisely defined, including restrictions on the environment around the query.
Two predefined substructures from the dictionary of >27,000 unique substructure queries matched against two different chemicals. The substructure is defined in detail, and, for example, atom 3 in the alkene, 1,1-disubst is closed to substitution, meaning that no additional bonds except those with hydrogens are allowed.
This dictionary of named substructure queries was matched against all chemicals in the dataset using the Leadscope software (13). A fingerprint table was generated for each compound, as illustrated in Figure 3. This example shows two chemicals from the dataset and a subset of the predefined named substructure queries. When a substructure query matches the chemical, it is marked with a “1”. The absence of a matching substructure query is marked with a “0”. In the example (Figure 3), both chemicals contain the feature “bicyclo[2.2.1]heptane”, whereas the first structure only contains “alkene, 1,1-disubst” and the second structure only contains “alkene, monosubst”.
Fingerprint table showing how two example chemicals are analyzed based on the predefined substructure queries (e.g., azulene, bicyclo[2.2.1]heptane, etc.), with 1 representing the presence and 0 the absence of a given substructure.
To support the grouping of the chemicals, it is important to be able to calculate the similarity between pairs of chemicals, meaning the similarity between the two corresponding binary vectors exemplified in Figure 3. A Tanimoto similarity score is calculated based on the matching substructures (14). This score uses the counts of the number of common matching substructures as well as substructures unique to each chemical, based on the following formula:
To illustrate this concept, the Tanimoto similarity score using the two chemicals and the small subset of the substructure queries shown in Figure 3 would be:
The Tanimoto similarity score ranges from 0 (least similar) to 1 (most similar), and it is dependent on the substructures used to represent the binary vector.
All pairs of chemicals in the dataset are compared based on this distance (i.e., 1−Tanimoto similarity) to identify the most similar pair of chemicals. After this initial pass, this pair is represented visually as shown in Figure 4 (step 1) where the length of the horizontal line is proportional to the calculated distance. In the next pass, the chemicals that have already been clustered (as shown in Figure 4 [step 2]) will not be considered individually and instead a representative distance to a member of the cluster is used. In the clustering approach of the present work, the maximum distance between a chemical and the chemicals in the cluster (referred to as complete linkage) was used. In Figure 4 (step 2), the next most similar chemical to the pair already identified is included and grouped with the horizontal distance representing the distance between the newly added chemical and the cluster containing the two chemicals. A dendrogram is progressively generated where all chemical structures are compared to both other structures as well as other clusters until all chemicals are included in the final analysis. This approach to clustering chemicals is referred to as agglomerative hierarchical clustering (14, 15). The dendrogram represents the relationship between all chemicals in the dataset. To generate a series of distinct groups, a cluster distance threshold needs to be established. This is illustrated in Figure 4 (step 3), where a distance is specified that results in two clusters. The default distance incorporated in Leadscope’s “Generate heat map” tool was used (13). This distance is calculated based on half of the distance of the “all compounds” cluster. It uses Complete Linkage (14), so a cluster’s distance is considered the maximum distance between the compounds, that is, the distance between the two most disparate compounds in the group. Once the clusters have been formed, they are examined manually and in some cases the grouping is modified based on additional expert considerations.
A pair of chemicals is initially grouped (Step 1), followed by an additional chemical in Step 2, then two groups are formed by specifying a cluster distance threshold.
2.3. Physicochemical Properties
Three calculated physicochemical properties were generated for all SAR-forms of the chemicals in the combined ELSIE and PQRI dataset: molecular weight, aLogP, and polar surface area (13). The frequency distribution of these three properties was plotted for both the ELSIE and the PQRI datasets.
2.4. Prediction Models
The resulting SAR forms of the combined dataset were analyzed for predicted mutagens and potent dermal sensitizers using in silico models and other sensitization predictions (i.e., moderate or weak sensitizers) were not considered in this analysis.
2.4.1. In Silico Evaluation for Mutagenicity:
In silico models are routinely used in the assessment of the DNA-reactive mutagenicity of E&L aligned with ICH M7 recommendations (8) of two complementary methodologies (expert rule-based and statistical-based). The Leadscope Bacterial Mutation expert alerts v8 (11) and Leadscope Bacterial Mutation statistical-based QSAR model v2 (16) were used to assess the E&L dataset. Experimental data were used directly; however, in the absence of any data, predictions from the two methodologies were used to generate a consensus mutagenicity call. Positive results in one or both methodologies from experimental data or in silico predictions were considered a flag for mutagenicity; however, all positive predictions were reassessed based on an expert review following standard documented procedures (17). Negative results in both methodologies were not considered a flag for mutagenicity. In the case of inconclusive results (e.g., indeterminate results or out-of-domain results), an expert review was performed on the results to assess whether the chemical contained a flag for mutagenicity. This review consisted of an interrogation of model features, an assessment of structurally similar analogs, and a review of reaction mechanisms.
2.4.2. In Silico Evaluation for Dermal Sensitization:
Currently, there is no specific guideline or regulation for the use of in silico models for dermal sensitization in E&L assessments; however, the use of sensitization models is outlined in a variety of publications (2, 5, 7). Similar to guidance for the application of in silico approaches in the evaluation of mutagenicity, statistical- and expert rule-based methodologies were used to predict dermal sensitization hazard (binary endpoint) and potency (ordinal endpoint). Based on the local lymph node assay (LLNA) EC3 value, European Centre for Ecotoxicology and Toxicology of Chemicals (ECETOC) potency predictions were utilized, where the EC3 is the amount of chemical inducing a threefold increase in lymph node cell proliferation compared with vehicle in the LLNA (Table I) (18).
Dermal Sensitization ECETOC Potency Categories
Training and reference sets of the statistical model (LLNA statistical version v3) and expert alerts model (LLNA alerts version v2) consist of LLNA data derived from the National Toxicology Program (NTP) Interagency Center for the Evaluation of Alternative Toxicological Methods (NICEATM) dataset and European Chemicals Agency (ECHA) records including 843 structures. The database that could be accessed for experimental data searching/analogs to support an expert review consists of 1528 structures. A consensus call was derived based on encoded rules that define various combinations of the predictions from the statistical and alert sets. Generally, the consensus is conservative, and a positive consensus call is generated if either the statistical or alert result is positive. The potency predictions are assigned based on the statistical model results. Only strong or extreme results from experimental data or predictions were considered to be of concern (flags with regards to E&L) (5). An expert review for both the chemicals predicted strong or extreme as well as inconclusive results (i.e., indeterminate or out-of-domain results) was performed, and compounds were recategorized in some cases with sufficient supporting information. Other predictions (i.e., moderate, weak, or nonsensitizers) were not considered flags. This approach is aligned with the framework described in Parris et al. (5).
3. Results
Both the ELSIE and PQRI compound datasets contribute to defining the E&L chemical space. Although there were 219 structures common between the two sets, the PQRI and ELSIE sets provided 370 and 194 unique structures, respectively (as illustrated in Figure 5) for a total of 564 unique structures.
Overlap in the PQRI and ELSIE datasets. ELSIE, Extractables and Leachables Safety Information Exchange; PQRI, Product Quality Research Institute.
In Figure 6, a portion of the clustering analysis of the combined ELSIE and PQRI datasets is shown. The dendrogram on the left shows the relationships between the chemicals based on their structural similarity, with the length of the horizontal lines proportional to the distance between the chemicals and the clusters. The list of chemicals is illustrated using their unique Leadscope identifiers (e.g., LS-1826). Information on whether each of the chemicals is present in the ELSIE dataset only, the PQRI dataset only, or both the ELSIE and PQRI datasets is shown as a heatmap (using red to represent the selected source(s)). The four remaining columns of the heatmap show whether there is an in silico flag for bacterial mutagenicity and/or strong or extreme dermal sensitization potential. It should be noted that for the clusters shown in Figure 6, there are no chemicals predicted as either bacterial mutagens or strong or extreme dermal sensitizers. The clusters formed are divided by white lines based on the selected cutoff distance (as discussed in Section 2.2), and the structures of the chemicals in the groups are also presented. For the 783 substances of the combined ELSIE and PQRI dataset, 314 groups were generated in total, including 172 singletons (clusters with only one chemical) based on the cluster analysis and expert review. The full dataset and results are shown in the Appendix. Out of the 142 chemical classes (containing two or more chemicals), 8 classes contained only chemicals from the ELSIE dataset, 29 classes contained only chemicals from the PQRI dataset, and the remaining 105 classes contained chemicals from both datasets. The Appendix contains the full dataset grouped by the classes generated.
Portion of the agglomerative hierarchical clustering of the combined ELSIE and PQRI datasets. ELSIE, Extractables and Leachables Safety Information Exchange; LLNA, Local Lymph Node Assay; PQRI, Product Quality Research Institute.
The bacterial mutagenicity and the dermal sensitization models were run for all compounds in the combined dataset, and an expert review was performed, as described in Section 2.4. The automated consensus bacterial mutation call included 69 positives, 645 negatives, and 69 inconclusives (which include indeterminate results and chemicals identified as out-of-domain); however, after an expert review, there were 69 positives, 712 negatives, and only 2 inconclusives. The automated LLNA consensus call included 26 strong or extreme dermal sensitizers, 520 not potent dermal sensitizers (i.e., nonsensitizer, weak, or moderate), and 237 inconclusives (which includes indeterminate results and chemicals identified as out-of-domain); however, after an expert review, there were 25 strong/extreme dermal sensitizers and 758 compounds that were not strong or extreme sensitizers. This expert review included a review of different alkanes that were identified as out-of-domain but which could be reassigned as nonmutagenic or nonsensitizers because they did not include any reactive structural features (i.e., high potency category according to Roberts et al. [19]). Three monographs performed as part of this expert review are included in the Appendix.
Table II summarizes the most populated groups, showing those clusters with ten or more examples. It includes branched and linear alkanes along with a number of groups containing a single functional group (e.g., aliphatic alcohols, aliphatic carboxylates). Other clusters include more complex congeneric chemical series (e.g., phthalates, (4-hydroxyphenyl)propionate derivatives). All classes (with the exception of large cyclosiloxanes) contain examples from both the ELSIE and PQRI dataset. Across these chemical groups, the prevalence of predicted mutagenic is approximately 2%, and there are no strong/extreme dermal sensitizers identified.
Summary of Chemical Classes with 10 or More Examples as Derived from Cluster Analysis
Table III represents the most common chemical classes that are unique to each dataset. For example, large cyclosiloxanes are unique to the ELSIE dataset, whereas substituted 1,3,-benzothiazoles are unique to the PQRI dataset. Isothiocyanates and alkyl bromides are unique to the PQRI dataset and include five chemicals (out of 60 included in Table III) that flag for mutagenicity and/or potent dermal sensitization. It should be noted that the three compounds reported under the class name 1,4-Dioctadecyl 2,3-bis[[3,5-bis(1,1-dimethylethyl)-4-oxo-2,5-cyclohexadien-1-ylidene]methyl]butanedioate are identical but for chirality: the first two are diastereoisomers (RS and RR) and the third does not specify the chirality of the two centers.
Comparison of ELSIE and PQRI Datasets Showing the Most Common Chemical Classes, Derived from a Cluster Analysis, That Are Unique to ELSIE and PQRI
The chemicals predicted to be mutagenic are summarized in Table IV and grouped by their respective primary alert. Seven alerts matching three or more examples are shown in the table, along with an indication of which dataset the chemicals were from. Similarly, Table V includes the compounds that alerted as potential potent dermal sensitizers.
Main Alerts Fired for Bacterial Mutagenicity with More than Three Examples
Main Alerts Fired for Strong/Extreme Dermal Sensitization
Figure 7 shows the frequency distribution of three properties (aLogP, molecular weight, and polar surface area) across all compounds in the ELSIE and PQRI datasets. Generally, the ELSIE and PQRI datasets had a similar profile. There is, however, a higher number of chemicals with low polar surface area in the PQRI dataset, which is reflective of the number of alkanes. Additionally, there is an elevated number of chemicals in the PQRI set with aLogP values between 1 and 3, which is reflective of several monofunctional chemicals (as illustrated in Table II).
Frequency distribution of physicochemical properties (aLogP, molecular weight, and polar surface area) across chemicals in the ELSIE and PQRI datasets. ELSIE, Extractables and Leachables Safety Information Exchange; PQRI, Product Quality Research Institute.
Figure 8 summarizes 74 chemicals predicted to be positive for bacterial mutagenicity and/or predicted to be a potent dermal sensitizer. This includes 49 chemicals that are predicted mutagenic (and not potent dermal sensitizers), 20 predicted both mutagenic and potent dermal sensitizers, and 5 predicted as potent dermal sensitizers (and not mutagenic). Four chemicals out of the 5 (106-51-4, 1948-33-0, 85-44-9, and 106990-43-6) were confirmed as strong or extreme dermal sensitizers following expert review and presented in an ELSIE monograph (5); 542-85-8 was flagged by alert #32 (Iso(thio)cyanates) and an expert review of this chemical is included in the Appendix.
Venn diagram of overlapping alerting structures.
For chemicals predicted to be mutagenic, 29% (20 out of 69) are also predicted to be potent dermal sensitizers. For chemicals predicted to be potent dermal sensitizers, 80% (20 out of 25) are also predicted to be mutagens. A separate analysis was performed of the Leadscope database to understand whether this trend is consistent across chemicals in general (13). The Leadscope database contains 21,180 chemicals with bacterial mutagenicity data and 966 chemicals with LLNA potency categories; 266 chemicals had both bacterial mutagenicity data and LLNA potency data. Of the 91 mutagenic chemicals (from the set of 266 chemicals), 32% (30 out of 91) are also potent dermal sensitizers (61 chemicals were mild, moderate, or nonsensitizers), whereas of the 50 potent dermal sensitizers, 60% (30 out of 50) are also mutagenic.
An analysis was performed of reaction domain categories, based on the Leadscope reaction domain profiler (v2) (13). Most of the chemicals that are flagged as mutagens and potent dermal sensitizers belonged to the second-order nucleophilic substitution (SN2) reaction domain (either pro- or direct) (11 out of 20) and the Schiff base formation (SB) reaction domain (5 out of 20). The pro-SN2 domain is represented by polycyclic aromatic hydrocarbons, which can be assigned to the high potency category for dermal sensitization according to the criteria described by Roberts et al. (19). There was no discernable trend related to reaction domain membership for the five chemicals that were flagged as nonmutagens and potent dermal sensitizers, although the small sample size precludes a thorough analysis of the correlation between the reaction domain, mutagenic, and sensitization potential. Figure 9 summarizes the distribution of the chemicals that were not flagged as well as those predicted to be mutagenic and potent dermal sensitizers across the two sources, PQRI and ELSIE.
Venn diagram with overlap between the sources (ELSIE and PQRI) and predictions (mutagens and strong/extreme dermal sensitizers). ELSIE, Extractables and Leachables Safety Information Exchange; PQRI, Product Quality Research Institute.
4. Discussion and Conclusions
This paper describes a chemical structural analysis of a representative set of E&L from the ELSIE and PQRI datasets containing 783 unique chemical structures in total. Around 74% of the chemical classes identified in the combined dataset included examples from both ELSIE and PQRI, and the most populated classes included linear and branched alkanes, carboxylic acid derivatives (esters and carboxylic acids), and aliphatic alcohols. There were, however, a few classes unique to each data source. For example, large cyclosiloxanes uniquely belonged to the ELSIE dataset, and isothiocyanates were contained only in the PQRI dataset. Some of these differences are explained by the scope of the PQRI dataset, which includes chemicals that have been known to extract or leach from manufacturing equipment. For example, isothiocyanates may be occasionally detected with extractable studies under specific conditions and may be associated with coatings, active films, and adhesives, where they may be incorporated as antimicrobial agents. Isothiocyanates are not observed as leachable substances.
The use of in silico models that predict bacterial mutagenicity and potent dermal sensitizers is considered a useful step in assessing the potential hazards associated with E&L to support determining safety thresholds. An in silico assessment using multiple complementary methodologies followed by an expert review of the results is considered best practice when incorporating computational toxicology methods into toxicological hazard assessments. The expert review in this case was able to refute practically all inconclusive predictions. The results of our evaluation showed that, of the combined ELSIE and PQRI datasets, around 9% (69 out of 783) of chemicals were flagged as potential mutagens and around 3% (25 out of 783) were flagged as potent dermal sensitizers. These observations support the findings by Parris et al. (5) that there is a low prevalence of potent dermal sensitizers in the E&L chemical space.
The most common mutagenic alerts observed included polycyclic aromatic hydrocarbons, unhindered epoxide, and primary aromatic amine. The alerts that flagged the most potent dermal sensitizers included polyaromatic hydrocarbon, iso(thio)cyanates, and aromatic diamino, dihydroxy-, or amino-hydroxy- compounds. The structural analysis identified no chemical classes unique to the ELSIE dataset in which any of the chemicals were flagged as a mutagen or potent dermal sensitizer. Only two chemical classes (each with three chemicals) unique to the PQRI dataset contained members predicted to be mutagenic and/or potent dermal sensitizers: alkyl bromides and isothiocyanates. In the alkyl bromide class, 2 out of the 3 chemicals were flagged as potential mutagens. It should be noted that acceptable limits may be derived for this class, as discussed in Bercu et al. (20). As discussed earlier, isothiocyanates have been occasionally observed within extractable studies of packaging material. Specific limits for food contact materials and for occupational safety and health for these chemicals have been established and may support any assessment of this chemical class.
This analysis showed that most (80%; 20 of25) E&L predicted to be strong or extreme dermal sensitizers were also flagged as potential mutagens. This is consistent with the analysis of the larger dataset, which indicated that the majority of potent sensitizers are mutagenic. In the combined dataset of E&L, there were only five predicted potent dermal sensitizers that did not alert for mutagenicity.
Based on the most common chemical classes derived from the cluster analysis of this representative dataset, further work could be performed to establish potential chemical class-based limits.
Conflict of Interest Declaration
The author(s) declare that they have no conflict of interests.
Acknowledgements
The authors wish to thank Scott Miller and Dave Bower from Instem for their technical support of this project and members of the ELSIE sensitization workstream for their valuable input.
APPENDIX
Phenanthrene (CASRN 585-01-8) Sensitization Monograph
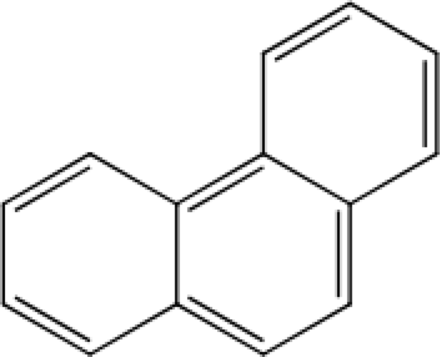
Potential for Human Exposure
Phenanthrene occurs as a major component of the total content of polynuclear aromatic compounds in the environment. Human exposure to phenanthrene occurs primarily through the smoking of tobacco, inhalation of polluted air, or by ingestion of food or water contaminated by combustion effluents (1).
Irritation
No dermal or ocular irritation studies conducted to current test standards could be identified for phenanthrene. Following repeat dermal administration carcinogenicity studies, phenanthrene was not irritating (2).
Sensitization
An in silico assessment of phenanthrene was performed using the Leadscope LLNA statistical-based model (v3) and expert alerts (v2) using the Leadscope model applier (v3.1). The LLNA consensus prediction was Strong/Extreme and the expert alerts fired alert #53 (Polyaromatic hydrocarbon).
No in vivo sensitization studies conducted to current test standards could be identified for phenanthrene. Old et al. (3) reported no contact sensitization to phenanthrene in guinea pigs. Phenanthrene was dissolved in complete Freund's adjuvant and emulsified with saline. Each animal was immunized in each front foot pad. Two to three weeks following immunization, each guinea pig was tested for contact sensitivity by applying one drop of a serial 10-fold dilution of phenanthrene dissolved in an acetone:olive oil mixture to the shaved ventral or dorsal skin. The reactions were recorded 24 h later.
In tests of polycyclic aromatic hydrocarbons (PAHs) that are present at relatively high concentrations in crude oils, phenanthrene did not induce photosensitization of mouse skin in vivo and in vitro (2).
No human case reports for dermal or respiratory sensitization were identified.
Regulatory and/or Published Limits
Phenanthrene is not classifiable as to its carcinogenicity to humans (Group 3) by International Agency for Research on Cancer (IARC) (1). The Research for Man and Environment (RIVM) published an oral tolerable daily intake (TDI) of 40 µg/kg/day for 17 PAHS, including phenanthrene, based on read across to the most sensitive hazard of benzo(a)pyrene exposure (4).
Expert Review
Based on the guinea pig contact sensitization test and repeat dermal administration studies in mice, phenanthrene is considered a nonsensitizer.
References
- 1.
- 2.
- 3.
- 4.
3-Vinylpyridine (CASRN 1121-55-7) Sensitization Monograph
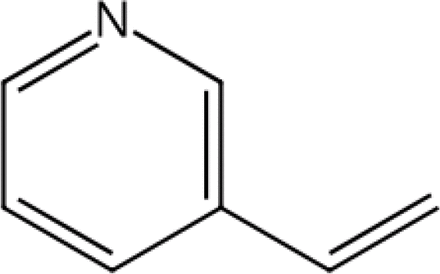
Potential for Human Exposure
3-Vinylpyridine is a smoke metabolite of nicotine (1).
Irritation
There are no irritation studies with 3-vinylpyridine available.
Sensitization
There are no sensitization studies with 3-vinylpyridine available.
The in silico assessment predicts 3-vinylpyridine as a strong/moderate sensitizer based on read across to 2- and 4-vinylpyridine, which are predicted and confirmed, respectively, as skin sensitizers via a Michael addition mechanism involving the polarized β-carbon:
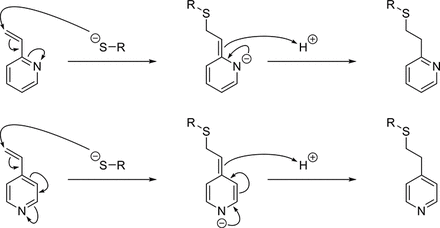
The Michael addition mechanism is relevant for 2- and 4-vinylpyridine, because the pyridine nitrogen has a stabilizing effect on the reaction intermediate. One robust LLNA study is available for 4-vinyl pyridine CAS 100-43-6, with an EC3 value of 1.6%, moderate potency (2, 3). This EC3 value has been extrapolated from the dose-response data and so bears the risk of overestimating the potency (4).
For 3-vinyl pyridine, the stabilizing effect is not possible given the position of the nitrogen with respect to the vinyl substituent, therefore preventing the Michael addition mechanism. This is confirmed by the lack of Michael addition structural alerts firing for 3-vinyl pyridine.
Regulatory and/or Published Limits
No regulatory or published limits are available for 3-vinylpyridine.
Expert Review
Based on the in silico and chemistry evaluation, including the lack of structural alerts and the less effective stabilization of the potential Michael acceptor intermediate by the pyridine nitrogen compared to 2- and 4-vinyl pyridine, 3-vinylpyridine is considered not to be a skin sensitizer.
Ethyl Isothiocyanate (CASRN 542-85-8) Sensitization Monograph
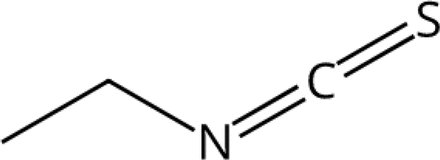
Potential for Human Exposure
Ethyl isothiocyanate (EITC) is used as a savory flavoring in the food industry, and Japan reports a daily intake of 0.03 µg/day (1). EITC has been implicated in causing allergic contact dermatitis from chloroprene rubber products. Diethylthiourea is used as an accelerator in the vulcanization process of chloroprene and is thermally degraded in the skin to EITC (2). EITC has shown some chemopreventive activity, although lower than that of phenethyl isothiocyanate (3, Chapter 6).
Irritation
In an OECD 439-compliant reconstructed human epidermis method, the mean viability of treated tissues was 2.4%. Therefore, EITC is considered irritating to skin (4).
In an OECD 438-compliant isolated chicken eye method, the cornea opacity score was 2.3; the fluorescein retention score was 3, and corneal swelling was 15%. Therefore, EITC is considered not irritating to the eye (4).
Sensitization
An in silico assessment of EITC was performed using the Leadscope LLNA statistical-based model (v3) and expert alerts (v2) using the Leadscope model applier (v3.1). The LLNA consensus prediction was Strong/Extreme, and the expert alerts fired alert #32 Iso(thio)cyanates and alert #39 (Cyanates, isocyanates and isotiocyanates).
In an LLNA, mice were treated with concentrations of 0.0008% to 1.6% EITC (vehicle not reported), which was applied to each ear, daily for three consecutive days. An EC3 of 0.04% was reported and corresponds to EITC being an extreme dermal sensitizer (2).
In a direct peptide reactivity assay (DPRA), ETIC caused complete peptide depletion at the first time point (2 min) and 7.8% depletion after 24 h (5).
Case reports of allergic contact dermatitis caused by chloroprene rubber have implicated EITC (2).
Regulatory and/or Published Limits
EITC has no safety concern at current levels of intake when used as a flavoring agent (1). EITC is also generally recognized as safe (GRAS) as a flavoring agent by Flavor and Extract Manufacturers Association of the United States (6).
Expert Review
Based on the LLNA, EITC is considered an extreme skin sensitizer.
Chemicals in the ELSIE and PQRI Combined Dataset Grouped by Chemical Class. The Cluster ID Represents the Different Classes, and the Cluster Size is the Number of Chemicals within Each Class
- © PDA, Inc. 2024